Photo credit: Shutterstock
As the world shifts toward greener energy sources, the renewable energy sector is poised for major growth – and fresh challenges.
Global policymakers set a target of tripling renewable energy capacity by 2030 at the COP28 climate change conference last year, and forecasts suggest that solar and wind power will lead the way.
Over the next five years, these two sources will account for 96% of capacity additions, according to the Renewables 2023 report from the International Energy Agency (IEA).
But it’s not all blue skies ahead.
Wind and solar energy sources are vulnerable to extreme weather conditions, putting energy management systems under increasing pressure and stretching traditional forecasting methods to their limits.
Enter Alibaba Group’s research institute, DAMO Academy.
A team of DAMO engineers and scientists is leveraging artificial intelligence and machine learning to improve the reliability of renewable energy forecasting and guide grid operators’ decisions about solar and wind power dispatch and real-time electricity balancing.
Their AI-backed weather prediction model has already been deployed in several provinces across China, which is at the forefront of solar and wind power development.
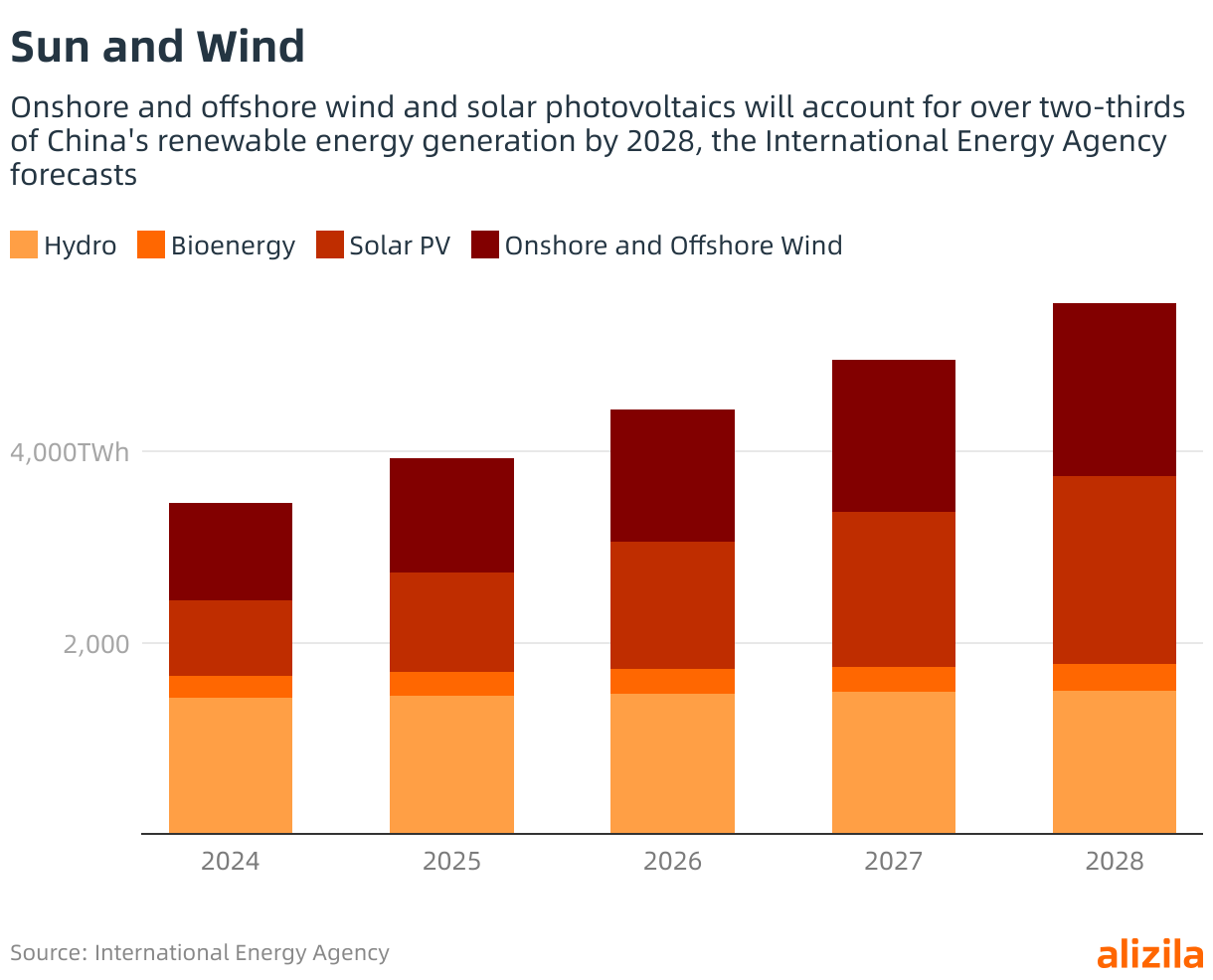
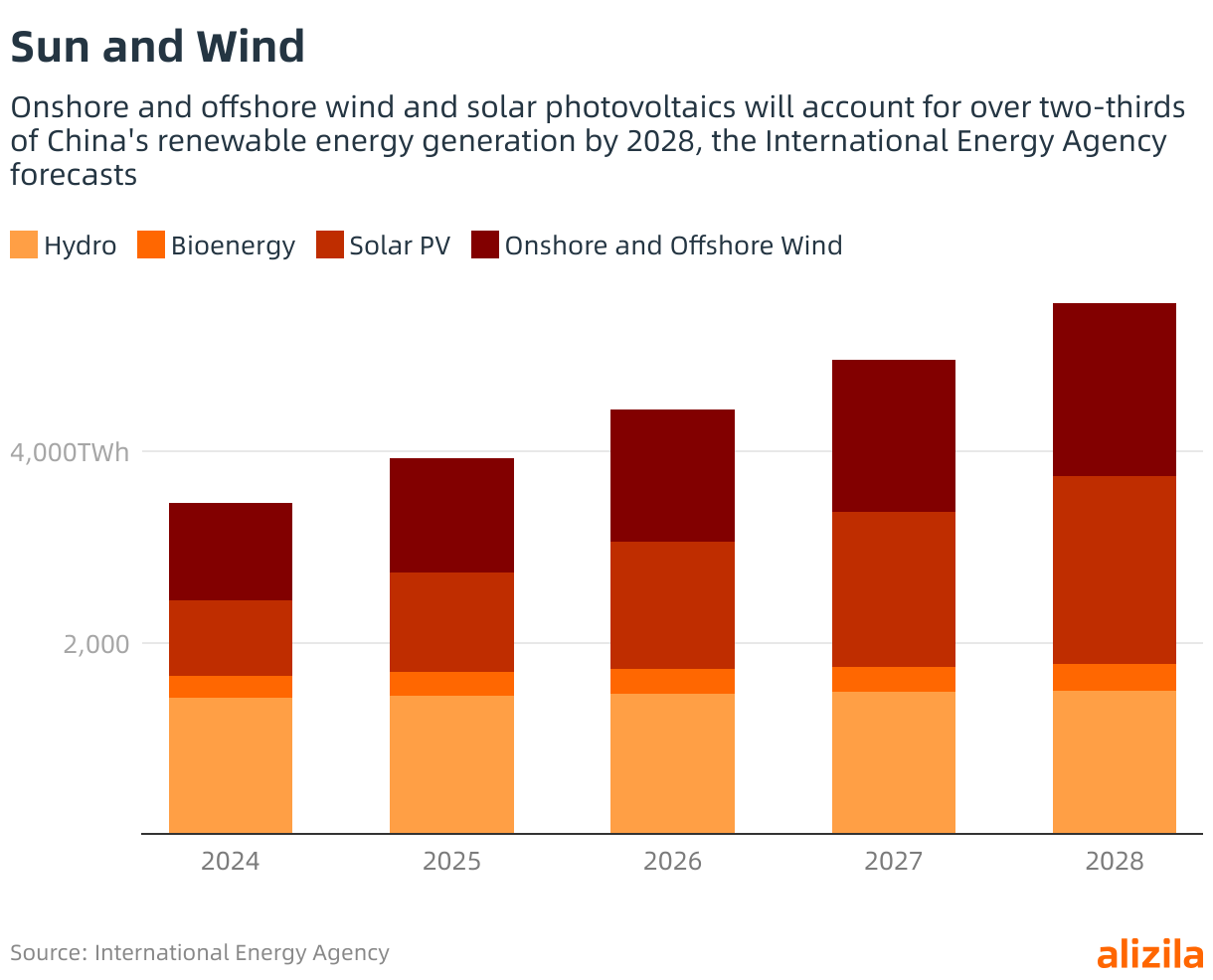
China commissioned the same volume of solar photovoltaics in 2023 as the entire world did in 2022, and grew its wind power capacity by 66% year-on-year, the IEA report shows.
To learn more about how AI technology could aid in these efforts and drive clean energy generation globally, Alizila spoke with Liu Le, senior product expert of DAMO’s Decision Intelligence Lab.
Below is a transcript of the conversation, edited for brevity and clarity
Q: Can you briefly explain what problems you’re solving in renewable energy prediction with AI?
A: A key challenge in renewable energy prediction is the inherent variability of wind and solar resources, which are highly dependent on fluctuating environmental conditions. Rapid changes in weather can lead to sudden shifts in energy production, making it hard to balance supply and demand, thereby risking damage to grid stability.
To minimize these impacts, we developed our renewable energy forecasting system with AI and machine learning technologies.
By integrating historical power output data, numerical weather forecasts, and real-time weather conditions, including critical variables such as temperature, wind speed, and solar radiation, our models generate highly accurate power predictions.
This enables reliable forecasts of wind and solar energy, allowing grid operators to make informed decisions regarding power dispatch and real-time electricity balancing. By effectively managing the intermittency of renewable energy sources, we help maintain grid stability and ensure a steady energy supply.
Q: What AI technologies does the system employ, and what are its standout features?
A: The system incorporates several cutting-edge technologies, particularly three key AI models: the power prediction model, weather prediction model and forecast interpretation model.
The power prediction model applies advanced machine learning algorithms, including deep learning techniques, to analyze vast datasets of historical energy production and weather conditions. It estimates how much energy can be generated from wind and solar resources at different times, thereby increasing the accuracy of forecasts.
The weather prediction model utilizes the public data from the European Centre for Medium-Range Weather Forecasts, which offers decades of historical insights into global weather conditions, combined with real-time data from Chinese weather forecast bureaus.
It employs a 2D-Vision Transformer framework, a scalable architecture for image processing tasks, that can easily integrate proprietary algorithm modules and accommodate large model parameters.
By combining high-quality meteorological data and innovative deep-learning architecture, the model aims to significantly improve forecast precision, addressing a key source of uncertainty in renewable energy generation.
The forecast interpretation model, an application of explainable AI, provides transparency by comparing historical data with current forecasts. This allows users to understand predictive discrepancies and move beyond black-box algorithms.
Our proprietary model offers the advantage of rapid processing and scalability, making it effective for large datasets. This transparency is crucial for building the trust of grid operators and energy experts, allowing them to validate the accuracy of our predictions and make informed decisions.
Together, these models enhance forecasting precision, improve grid operation safety, and ultimately support more effective energy management strategies.
Q: Can you share some successful case studies where this system has been implemented?
A: Currently, our renewable energy forecasting system is deployed in several provinces in China, such as Shandong, Zhejiang and Hubei, covering wind farms and rooftop photovoltaics.
In Zhejiang province, the average rate of unqualified forecasts among wind farms decreased from 20.7% to 8.4%. This unqualified rate represents the percentage of days within a month when forecasting accuracy drops below 85%, the standard assessment rate used by grid operators.
This notable improvement in forecasting enhances operational efficiency in power generation management and increases overall power grid safety. As a result, grid operators can balance supply and demand more effectively, reducing the risk of outages.
Through the explainable AI method, we’re also able to uncover the working mechanism of the predictive models, which leads to greater adoption and user satisfaction.
Q: What challenges did you face while developing this system?
A: We encountered numerous challenges during the development process. One significant hurdle was ensuring data quality and quantity; often, the data analyzed was either inadequate or insufficient for building reliable prediction models. Additionally, the variability of weather conditions posed another challenge since renewable energy generation is heavily influenced by environmental factors.
To address these issues, we integrated precise meteorological data, ensuring higher accuracy in our load and power predictions. Furthermore, we continuously work to enhance our AI models to adapt to newly emerging patterns in climate and energy use.
Q: Do you see the potential for broader use of this technology globally?
A: Globally, we envision this system can significantly enhance the safety and reliability of power systems by reducing uncertainties associated with renewable energy generation.
The ability to provide accurate forecasts allows regions to better respond to climate change challenges and supports the global transition toward sustainable energy solutions.
By equipping utilities around the world with these advanced forecasting capabilities, we aim to promote energy security and facilitate the integration of more renewable resources into existing power grids.
Our system has been highlighted in the AI for Good—Innovate for Impact report by the International Telecommunication Union, which showcases about 50 global AI for Good cases out of more than 200 submissions from 38 countries. This recognition encourages us to continue evolving the system.
Q: How will you continue to develop this technology?
A: We plan to continue advancing our technology by focusing on the development of enhanced weather models that further improve accuracy and reliability. We also aim to integrate expert knowledge in our predictive framework leveraging large language models, increasing the adaptability and precision of our forecasts.
Additionally, we are exploring the possibilities of applying the technology in more countries and regions to help them overcome their renewable energy forecasting challenges.
Through persistent innovation and technological optimization, our renewable energy forecasting system is positioned to make a meaningful impact on global clean energy solutions and effective electricity management.
For more sustainability news, click here