Photo credit: Shutterstock
The latest language model series from Alibaba Cloud topped rankings for open-sourced LLMs shortly after launching on Friday, thanks to its enhanced performance and improved safety alignment.
The Qwen2 model series encompasses a number of base language models and instruction-tuned language models with sizes ranging from 0.5 to 72 billion parameters, as well as a Mixture-of-Experts (MoE) model.
Its updated capabilities landed it first place on the Open LLM Leaderboard from the collaborative artificial intelligence platform Hugging Face, where it is available for commercial or research purposes.
“We hope to build the most open cloud in the AI era, making computing power more inclusive and AI more accessible,” said Alibaba Cloud’s Chief Technology Officer Zhou Jingren.
In addition, the Qwen2 models are available on Alibaba Cloud’s own AI model community ModelScope.
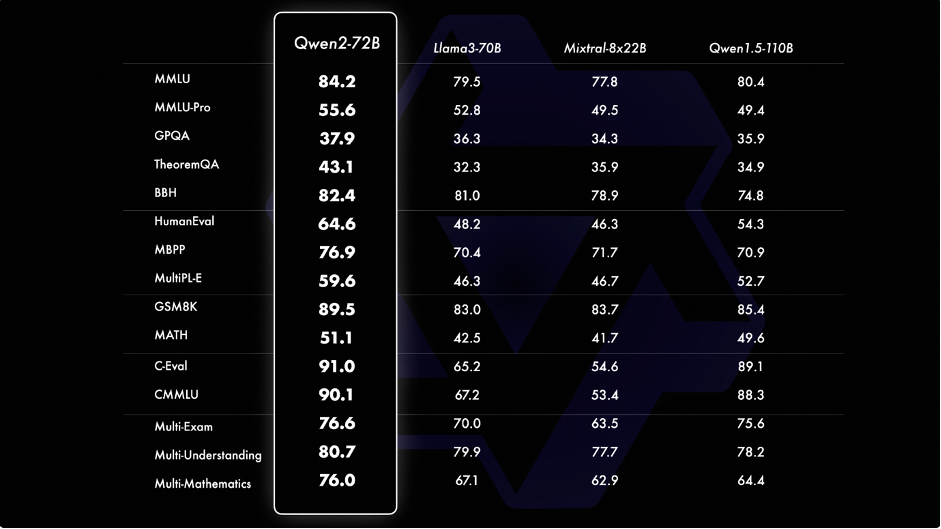
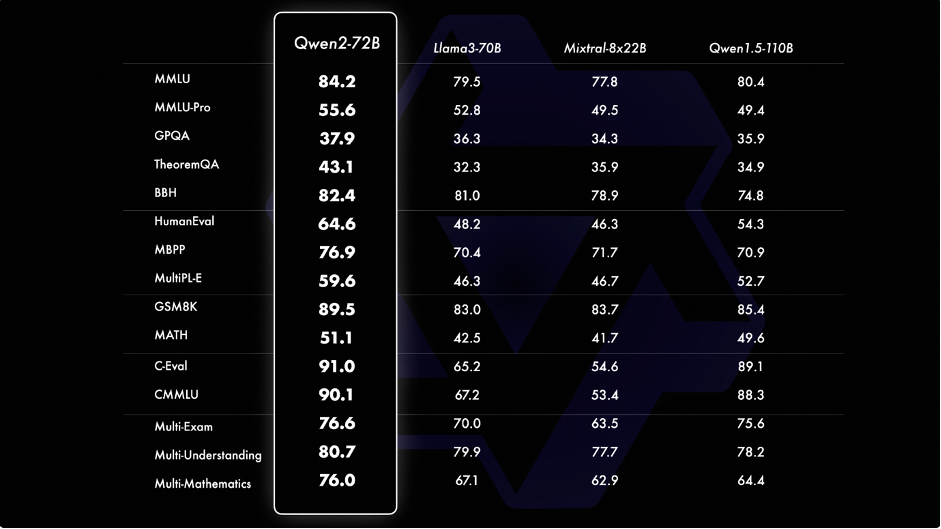
Enhanced Performance
Leveraging Alibaba Cloud’s optimized training methods, the large-size model Qwen2-72B model outperformed other leading open-source models in 15 benchmarks, including language understanding, language generation, multilingual capability, coding, mathematics and reasoning.
In addition, Qwen2-72B shows an impressive capacity to handle context lengths up to 128K tokens, the maximum number of tokens the model can remember when generating text.
To bolster their multilingual capabilities, 27 languages, in addition to Chinese and English, were included in the Qwen 2 training. These range from German and Italian to Arabic, Persian and Hebrew.
In addition, Qwen2 models boast increased speed while using less memory in model inference due to a technique called Group Query Attention, which optimizes the balance between computational efficiency and model performance.
Responsible AI
Besides being whizzes at math and linguistics, Qwen2 models’ output demonstrates better alignment with human values.
Comparative performance on benchmarks like MT-bench, a multi-turn question set that evaluates a chatbot’s multi-turn conversational and instruction-following ability, showed Qwen2 scored highly in these two critical elements for human preference.
By incorporating human feedback to better align with human values, the models have achieved good performance in safety and responsibility. They are capable of handling multilingual unsafe queries related to illegal activities like fraud and privacy violations to prevent the misuse of the models.
In terms of smaller models, Qwen2-7B also outshines other state-of-the-art models of similar sizes across benchmarks, including coding.